Intelligent Medical Coding
With 20–30% of terms requiring manual coding, CluePoints strives to cut the coding effort in half. Intelligent Medical Coding harnesses advanced Deep Learning (DL) technology to enhance the accuracy and efficiency of coding in clinical trials. It seamlessly integrates with existing systems to offer precise, AI-generated coding suggestions. This automation dramatically reduces the need for manual dictionary searches and costly coding reviews, freeing up valuable resources and ensuring uniformity across all coded data.
USERS
DE-RISKED
DETECTED
Deep Learning Model
Unlock unparalleled accuracy and efficiency in medical coding with advanced capabilities.
High-Probability Suggestions
Our Deep Learning (DL) model provides coding suggestions for adverse events and concomitant medications at up to 99% accuracy, streamlining manual coding.
Streamlined Processes
Significantly reduce the time and effort spent manually coding terms while also increasing coding consistency.
Improved Quality Control
Minimize manual quality control checks, reducing inconsistencies across medical coders and studies while saving time and resources.
Dictionary Upversioning
Our DL model can automatically handle regular upgrades of the WHODrug and MedDRA dictionaries, with up to 80% accuracy for completely new terms.
Broad Vocabulary Support
Ensure accurate coding across a broad spectrum of medical jargon, drug names, and emerging terminology.
Semantic Understanding
The DL model understands the meaning of medical terms, even for nuanced terms such as translating “ache” to “pain” effectively.
Automated Coding Processes
Traditional coding systems use synonym lists to help match terms from medical records to standardized medical codes. However, not all terms can be matched automatically. Medical coders spend considerable time assigning codes, typically with 85% accuracy on initial review. These are subject to secondary review, in which an approver accepts or rejects decisions, adding a layer of scrutiny and quality control before finalizing the medical code. Our DL model supports the manual coding process by providing suggestions for codes at up to 99% accuracy.
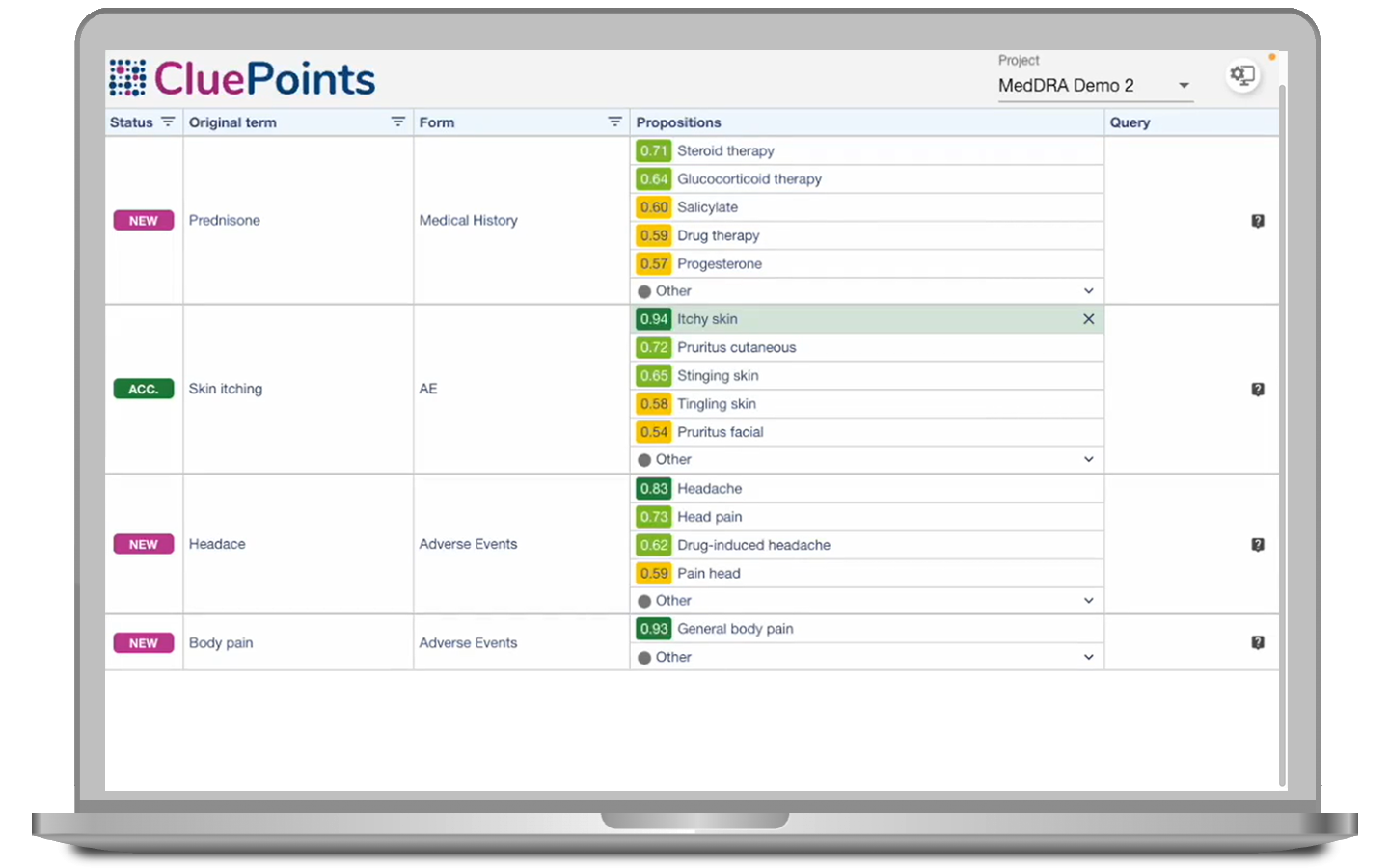
Continuous Improvement with DL
Our DL model leverages insights from auto-coding and the decisions of medical coders to continuously maintain and improve the suggestions sent for each verbatim. This advancement transforms coders’ roles from essential data management to more analytical data science tasks. Coders now focus more on approving, reviewing, and analyzing coding trends, which adds value by enabling deeper insights into clinical trial data.
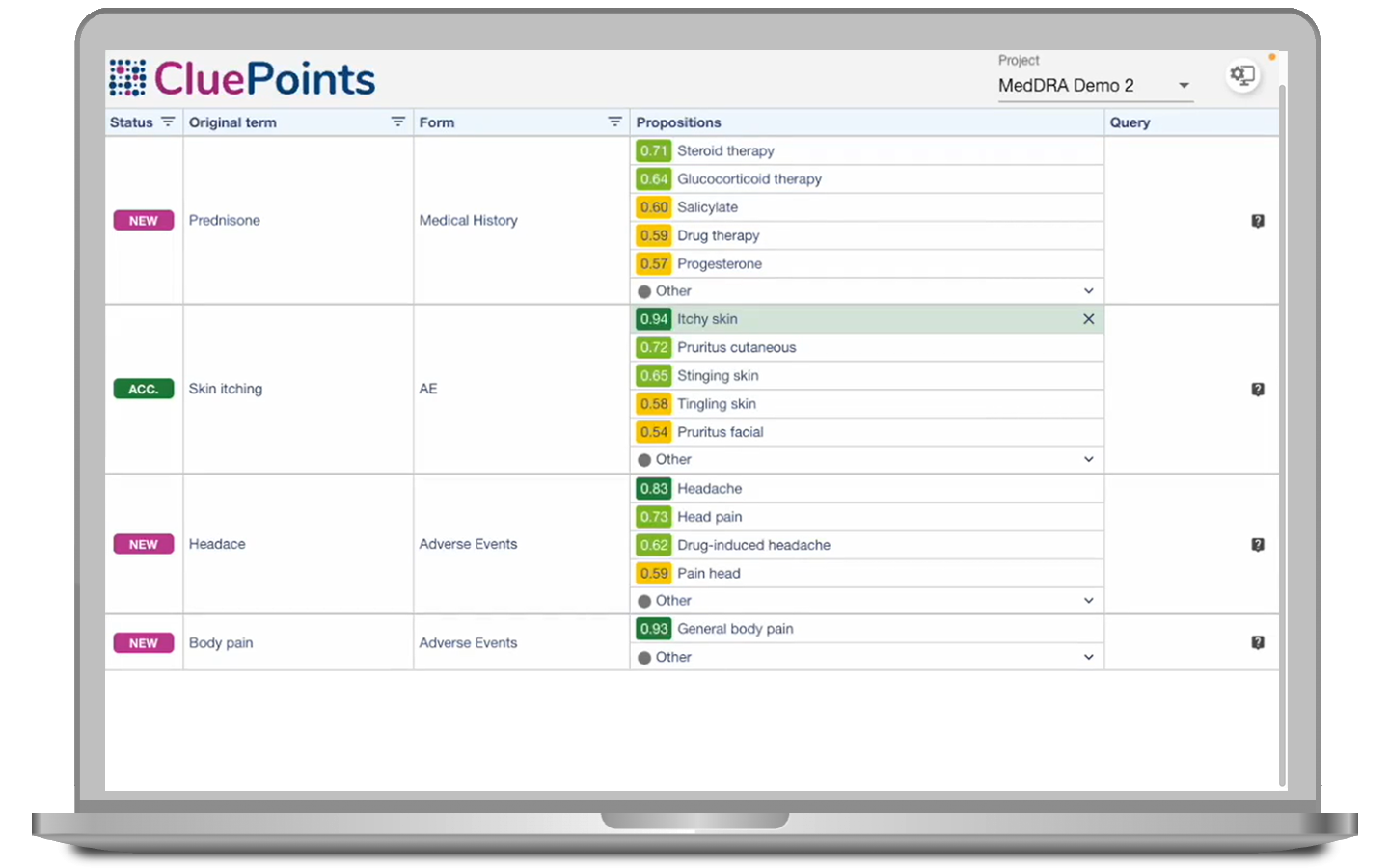
Quality Control Checks
Manual quality control checks in medical coding often result in inconsistencies due to human error, varying interpretations, and the sheer volume of data to be reviewed. These checks are time-consuming and prone to error, leading to potential inaccuracies in the coding process as well as additional time and cost for review. Our system enables risk-based quality control of medical codes, ensuring accurate coding with limited manual intervention. Through continuous learning and adaptation, our DL model minimizes errors and discrepancies, providing reliable results with unmatched consistency.
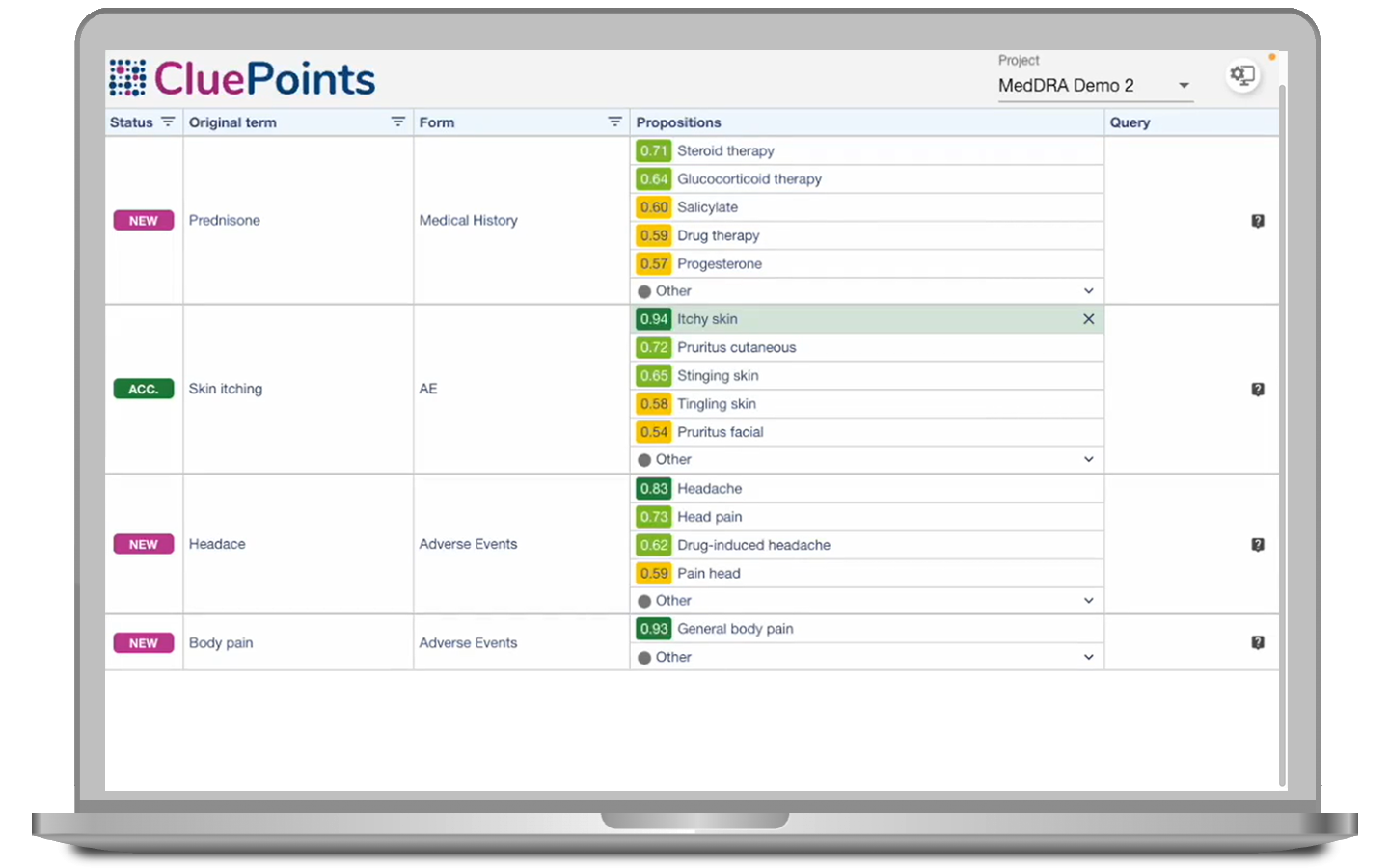
Implementation Support
CluePoints supports the implementation of our medical coding solution in ongoing clinical trials. Our professional services team is available to guide your journey every step of the way.
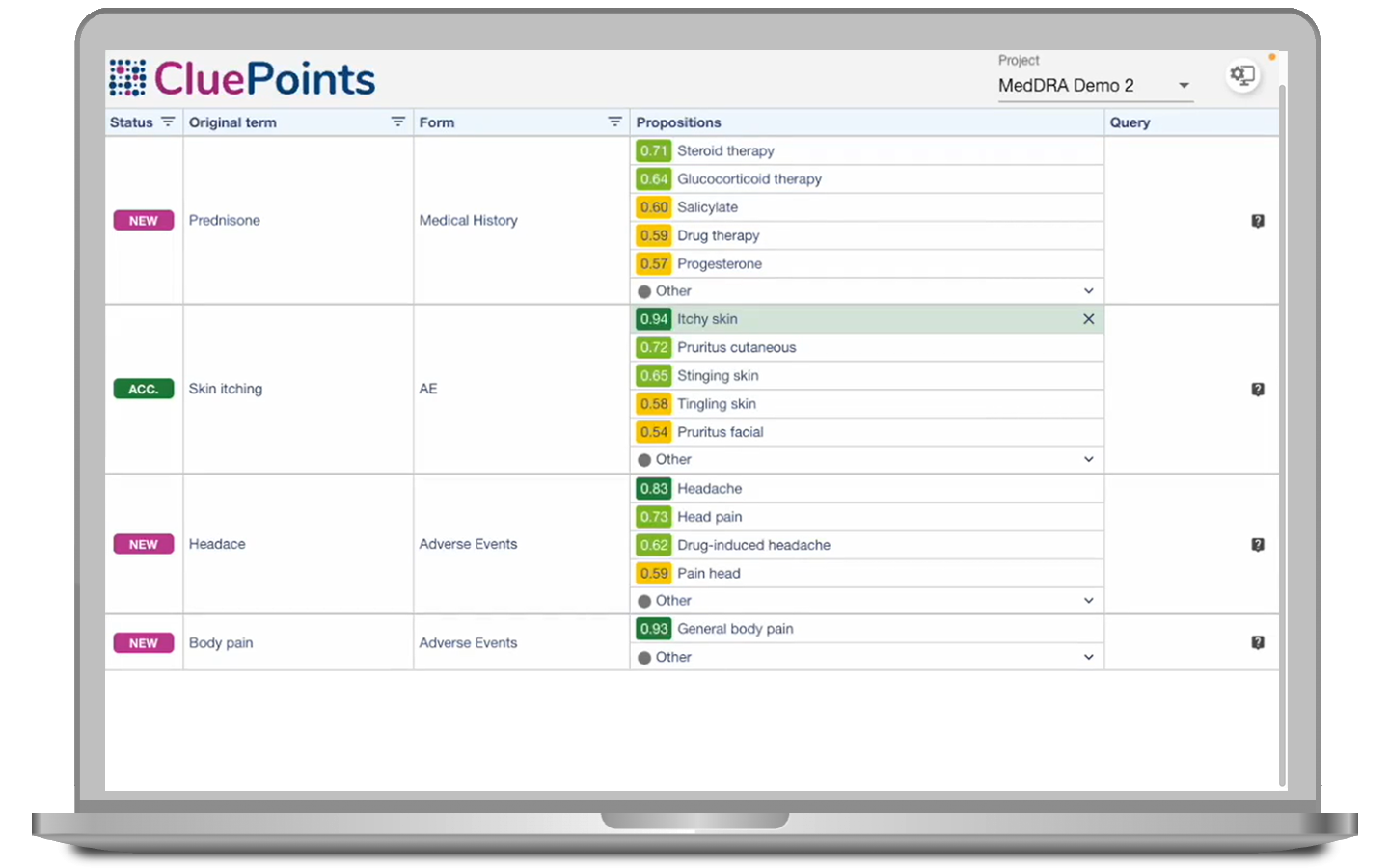
Explore Our Knowledge Center
Intelligent Medical Coding for Every Clinical Trial
CluePoints provides Sponsors and CROs with a smarter way to detect and manage risks that could impact clinical trial outcomes. By leveraging the potential of AI and advanced statistics, CluePoints is transforming a powerful, best-in-class platform of solutions into positive clinical development outcomes as our partners work to develop innovative therapies to improve the lives of people worldwide.
Contract Research Organizations (CROs)
Pharmaceuticals
Risk-Based Quality Management (RBQM)
In addition to medical coding, CluePoints’ RBQM solutions illuminate real-time anomalies through cutting-edge statistics and ML, offering users a superior method to manage trial risks and accelerate resolution.
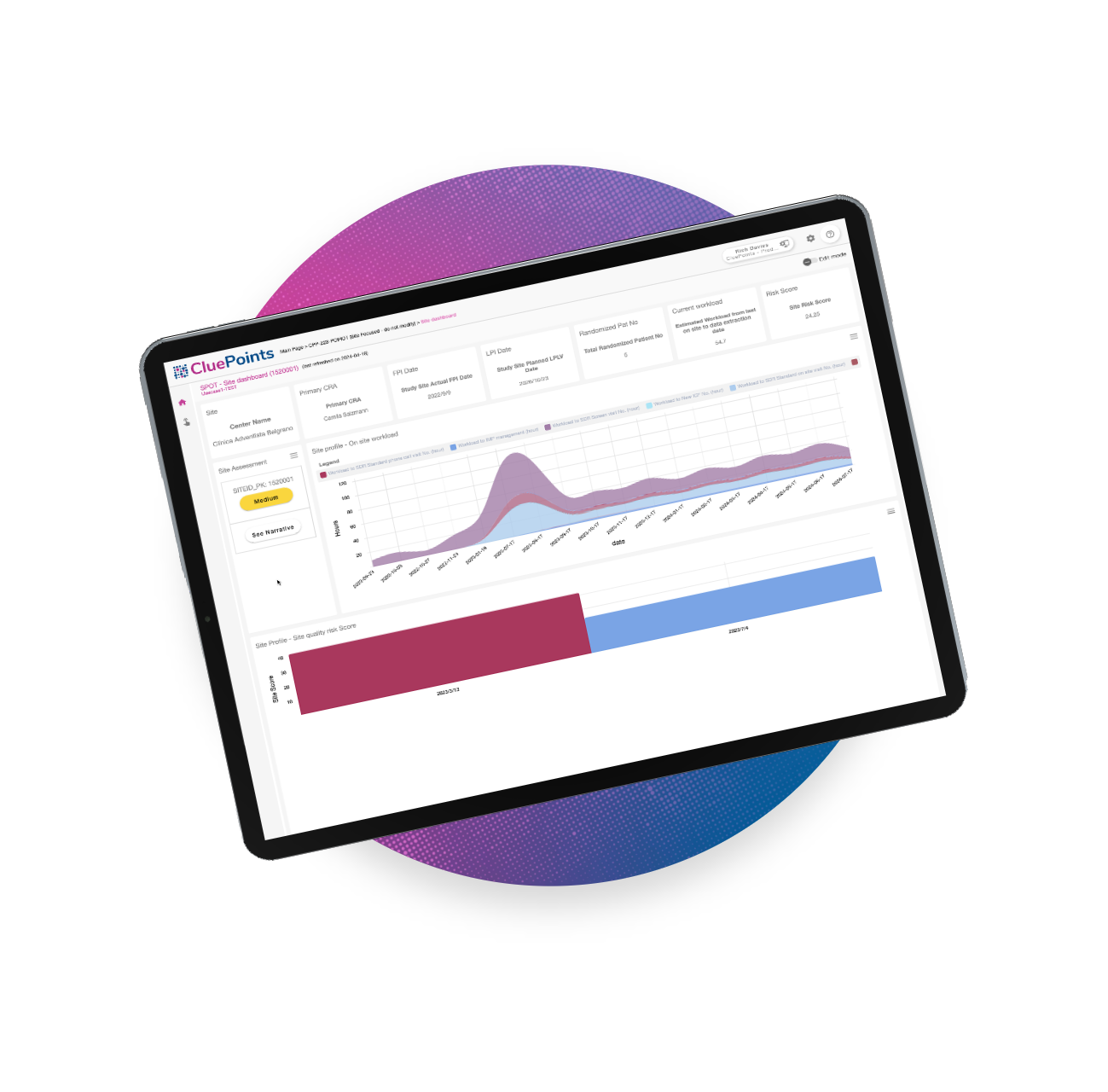